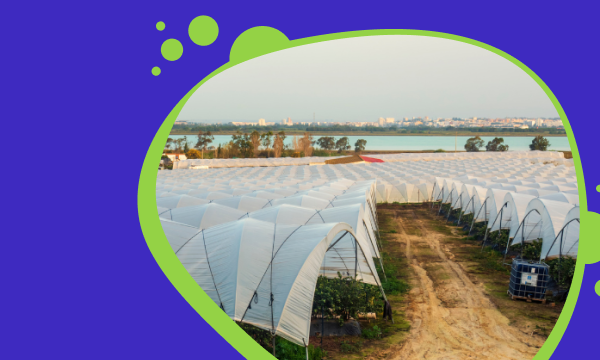
- WEBINAR
- CROP MANAGEMENT
4 common grower problems solved with digital agronomy
The protected crop growers guide to successfully managing your plants, team, practices and...
19.07.2023 | 35 min watch
For protected cropping growers, predicting yield is a crucial practice that drives both short-term response and long-term strategy. While many growers aim for a high level of yield prediction accuracy, they may not achieve it consistently.
Using data and insights from our Beyond Yield Prediction: The Importance of Climate and Context in Tomato Production whitepaper, this webinar presents the case for a more holistic approach to yield forecasting. Find out how interpreting yield prediction with additional contextual data improves decision-making and understand:
This webinar is presented by:
Welcome to today's webinar. We're going to cover three things. The first thing I'm going to go over is the singular focus on achieving high accuracy in yield prediction. Is this the right approach? While yield production is a great tool and we strive for high accuracy, we need to question if this is indeed the right approach.
Then, we'll discuss yield swings, specifically what they are and what causes them. Finally, we'll talk about interpreting your prediction with greater context to improve consistency and improve confidence in our outcomes.
This research, produced by Dr. Tharindu Weeraratne and Dr. Mpatisi Moyo, is also available in the Beyond Yield Prediction whitepaper.
We're covering yield prediction and forecasting today, but let's start with a quick refresher on why we do yield prediction and what we aim to achieve with it. Yield prediction drives long and short-term decision making. For long-term decision making, it's about ensuring we produce enough over the season to meet our contracts and revenue targets. In the short term, it's about steering our plants to meet our immediate contract requirements. Yield prediction allows us to understand when we might not meet our targets and enables us to steer our crop towards our desired outcomes. If we're going to produce too much or too little, we can adjust accordingly. Yield production increases our confidence in planning and fulfilling our contracts.
But how does yield prediction work? At WayBeyond, we use an AI-based machine learning model that utilizes historical data to find patterns and predict future outcomes. This model looks at patterns in yield and environmental conditions and applies them to current greenhouse conditions to forecast future yields. This approach is great, but it does have challenges. It requires a vast amount of historical data, which isn't always available or may be siloed. Furthermore, changes in climate and weather patterns can make historical data less relevant.
One significant issue is the variable accuracy of yield predictions. Sometimes our predictions are spot-on, other times, not so much. The accuracy can range from 65% to 100%, and it changes every week. This variable accuracy is a challenge across all methods of yield prediction.
So, we're all aiming for high accuracy in yield prediction, but with these challenges, is focusing solely on this accuracy the right approach? In this presentation, I'm going to argue for a holistic approach to planning and forecasting, where yield prediction is just one of the tools we use. It's important, but we should also consider what's going on in the environment, the plants themselves, and our management practices.
To understand the impact of variable accuracy, we looked at 20 cycles of tomato harvest data. We grouped these cycles based on average yield prediction accuracy and then analyzed the number of yield swing weeks in each group. We found that cycles with the lowest yield prediction accuracy had the most yield swings. This suggests that as the number of significant deviations from expected yields increases, the accuracy and usefulness of yield prediction decrease.
For both low and high-tech growers, various factors can influence these yield swings. Understanding these factors is crucial. We analyzed environmental data before low and high yield swing weeks and identified common factors such as low outside night temperature, total light, and internal day-night temperature differences that correlated with yield swings.
We also looked at plant data and found that plant state before a yield swing week is an important indicator. Before a low swing week, plants were often in a vegetative state, while before a high swing week, they were more generative.
So, what does this mean for tomato growers? Yield prediction is more effective when supported by relevant local insights. It's a great tool, but it should be used alongside understanding of the environment and plant conditions. Rather than solely focusing on maximizing prediction accuracy, it's more beneficial to understand the broader context of greenhouse conditions. Investigating your yield swings and trends can help anticipate and manage future production. Plant state is a crucial indicator of potential swings, so monitoring plant measurements is essential.
In conclusion, while yield prediction is an invaluable tool, it should be part of a holistic approach to planning and forecasting in protected cropping agriculture.
The protected crop growers guide to successfully managing your plants, team, practices and...
Learn how interpreting crop yield prediction with contextual data improves decision-making and...
SaaS adoption in horticulture: discover challenges and pitfalls for both growers and technology...
Discover the true cost of a fungal disease outbreak in crops, how to respond and the financial...
Get insights on Integrated Pest Management planning for effectively managing infestations.
Learn how to manage plant stress and improve crop performance with Vapour Pressure Deficit.
Plant pathology is the study of plant diseases and how they interact with plants. In this in-depth...
Champions of crop management.
Giving protected growers the power to make better crop management decisions and optimize crop outcomes.
Solutions
Customers
©2025 WayBeyond. All Rights Reserved.